Relevance workbench
In this workbench, you can compare our Elastic Learned Sparse Encoder model (with or without RRF) and traditional textual search using BM25.
Start comparing different hybrid search techniques using TMDB's movies dataset as sample data. Or fork the code and ingest your own data to try it on your own!
Try these queries to get started:
- "The matrix"
- "Movies in Space"
- "Superhero animated movies"
Notice how some queries work great for both search techniques. For example, 'The Matrix' performs well with both models. However, for queries like "Superhero animated movies", the Elastic Learned Sparse Encoder model outperforms BM25. This can be attributed to the semantic search capabilities of the model.
Explore similar demos
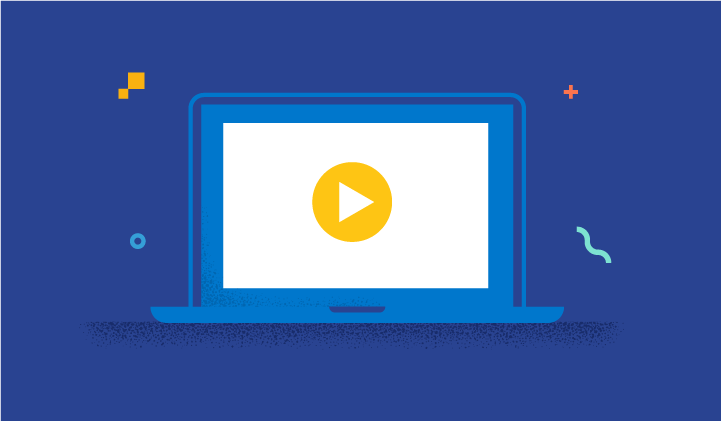
Search
Elasticsearch foundations
Get started with Elasticsearch in this short video series. Build your foundation by learning how to set up users, roles, and permissions; manage and move data with index lifestyle management (ILM) policies; and more.
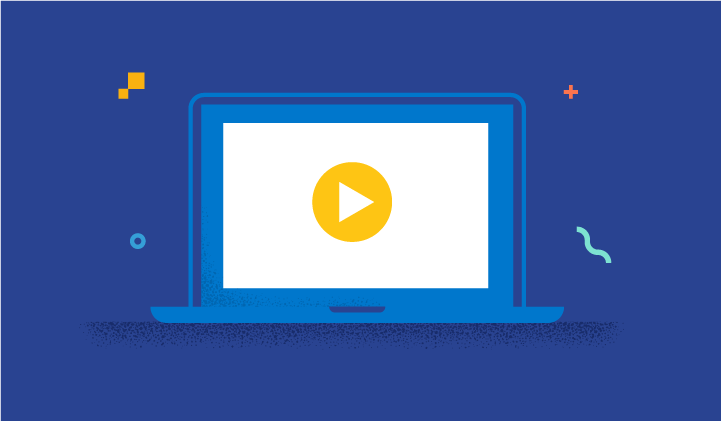
Search
Getting started with search applications
Ramp up on this short video series. Learn how to easily get started with Elasticsearch Serverless, how to generate vector embeddings with Elastic Learned Sparse EncodeR (ELSER), and more.
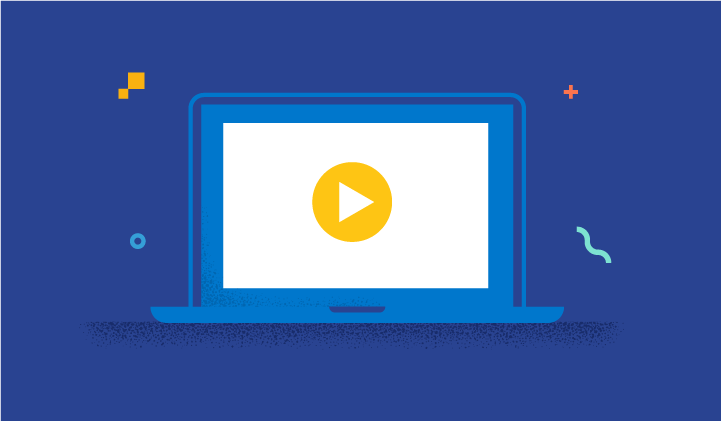
Security
Searching, alerting, and analyzing logs
Start building your foundation in Elastic Observability with this short video series. You’ll learn how to triage and isolate issues faster, monitor your infrastructure and applications, use logs and machine learning for quicker root cause analysis, and more — across your entire environment, at scale.