Reflecting on all of the changes surrounding speed, scale and relevance in Elasticsearch up through the latest 8.15.0 release, it's phenomenal how far we've come.
This post serves as an aggregation and timeline of all the great work that has been put into Elasticearch and Lucene to make it the best vector database. We dive into significant strides in Lucene vector search performance and other Lucene improvements if you want even more information about how it all works under the hood.
Elasticsearch 7.x
Our story started all the way back in Elasticsearch 7, when some of our visionary engineers advocated that vector search was important and we should support it.
Some of the changes in version 7 included introducing field types for high-dimensional vectors, vector similarity functions, and vector script functions for dense vectors to be used in brute force search.
This was only the beginning...
Elasticsearch 8.0
Elasticsearch 8.0 introduced native support for ANN search.
Additionally, Elasticsearch introduced experimental support for kNN search over HNSW for float vectors.
Elasticsearch 8.2
In Elasticsearch 8.2, we added filtering support to kNN search.
Elasticsearch 8.2 shipped with Lucene 9.1, which included kNN search with filtering, improved vector merge speed by reducing I/O, and faster l2_norm
calculations through unrolling and auto-vectorization.
Elasticsearch 8.3
Elasticsearch 8.3 shipped with Lucene 9.2, which improved HNSW graph performance by performing a diversity check of neighbors and correcting the base layer connection count.
Elasticsearch 8.4
In Elasticsearch 8.4, kNN search was made generally available and added to the _search
endpoint. This unlocked functionality such as hybrid search with filtering, reranking, and semantic search combined with lexical search.
Elasticsearch 8.5
In Elasticsearch 8.5, we added support for synthetic source for dense_vector
fields.
Elasticsearch 8.5 shipped with Lucene 9.4, which added support for byte encoded vectors, and optimized performance by building the hnsw graph during indexing to improve flush time.
Elasticsearch 8.6
Elasticsearch 8.6 added support for byte vectors, so that users can quantize vectors outside of Elasticsearch.
Elasticsearch 8.7
Elasticsearch 8.7 added the ability to search more than one kNN field at a time.
Elasticsearch 8.7 also shipped with Lucene 9.5, which came with improved HNSW graph storage efficiency.
Elasticsearch 8.8
Elasticsearch 8.8 was a huge release. Not only did we increase dimensionality support to 2048 for dense_vector
queries, but we also launched the text_expansion
query for sparse vector queries and the state of the art ELSER V1 model.
Elasticsearch 8.8 shipped with Lucene 9.6, which offered improved HNSW merge performance by reusing the largest graph.
Elasticsearch 8.9
8.9 was another huge release for performance gains, introducing SIMD support with the Panama Vector API.
On top of that, query_vector_builder
was made generally available, and RRF was introduced.
Elasticsearch 8.9 also shipped with Lucene 9.7, which added multi-segment parallelism to kNN queries, sped up brute force for floating point vectors by adjusting how they are encoded on disk, and sped up brute force search with vectors by utilizing the Panama Vector API.
Elasticsearch 8.10
In Elasticsearch 8.10 multi-segment search parallelism was introduced.
Elasticsearch 8.11
With Elasticsearch 8.11, we leveraged passage vector search support in Lucene to support passage vectors in Elasticsearch through nested fields. Additionally, we increased the maximum number of vector dimensions to 4096, and added support for maximum inner product. We reintroduced sparse_vector
field mapping, released a new, improved and generally available ELSER V2 model, and started indexing dense vectors by default (with dynamic mapping).
Elasticsearch 8.11 also leveraged Lucene 9.8, which added MIP support.
Elasticsearch 8.12
In 8.12, we launched kNN as a query, unlocking a huge amount of functionality within our query DSL. Additionally, we added the int8_hnsw
index type for auto-quantized vectors, improved cosine similarity performance by normalizing vectors, enabled query phase parallelism within a single shard, and added byte quantization for float vectors in HNSW.
Elasticsearch 8.12 also leveraged Lucene 9.9, which added multi-threaded graph building and an int8 scalar quantized format.
Elasticsearch 8.13
With Elasticsearch 8.13, we added int8_flat
for flat storage of auto-quantized vectors, enabled multiple inner-hits for passage vector search, and further simplified the knn
query by making k
and num_candidates
optional. Additionally, we launched token pruning to speed up sparse vector queries sparse models such as ELSER.
Elasticsearch 8.13 was shipped with Lucene 9.10, which included multi-segment HNSW graph search improvements.
Elasticsearch 8.14
Elasticsearch 8.14 included NEON SIMD optimized native index operations for int8_hnsw
indices, support for hex-encoded byte strings for byte vectors, defaulted dense vector fields to use int8_hnsw
, and further enhanced the kNN query builder by adding modelId
and modelText
.
Elasticsearch 8.15
And here we are at Elasticsearch 8.15 - a huge release that includes:
- Elasticearch 8.15 is now SIMD native optimized for index and search for
int8_hnsw
indices - Adds int4 scalar quantization using
int4_hnsw
andint4_flat
vectors - Adds support for bit vectors for both hnsw and flat vectors, and added hamming distance for brute force search
- Introduces a new
sparse_vector
query to search sparse vectors with inference endpoints or precomputed query vectors - Adds a new semantic_text field and semantic query to make it easier than ever before to start using semantic search!
Elasticsearch 8.15 also ships with Lucene 9.11, which also has a ton of great optimizations:
- Improved speed from Lucene madvise optimizations
- Adds a new
VectorScorer
interface for better brute force scoring - Includes optimized scalar quantiles to allow dynamic quantiles calculations
- Adds int4 scalar quantization & optimized SIMD code for int4 comparisons
... and beyond!
We're nowhere close to done, so make sure to check out all of the new features in 8.15 and serverless, and keep an eye on Search Labs to hear about all of our new features!
Ready to try this out on your own? Start a free trial.
Elasticsearch has integrations for tools from LangChain, Cohere and more. Join our advanced semantic search webinar to build your next GenAI app!
Related content
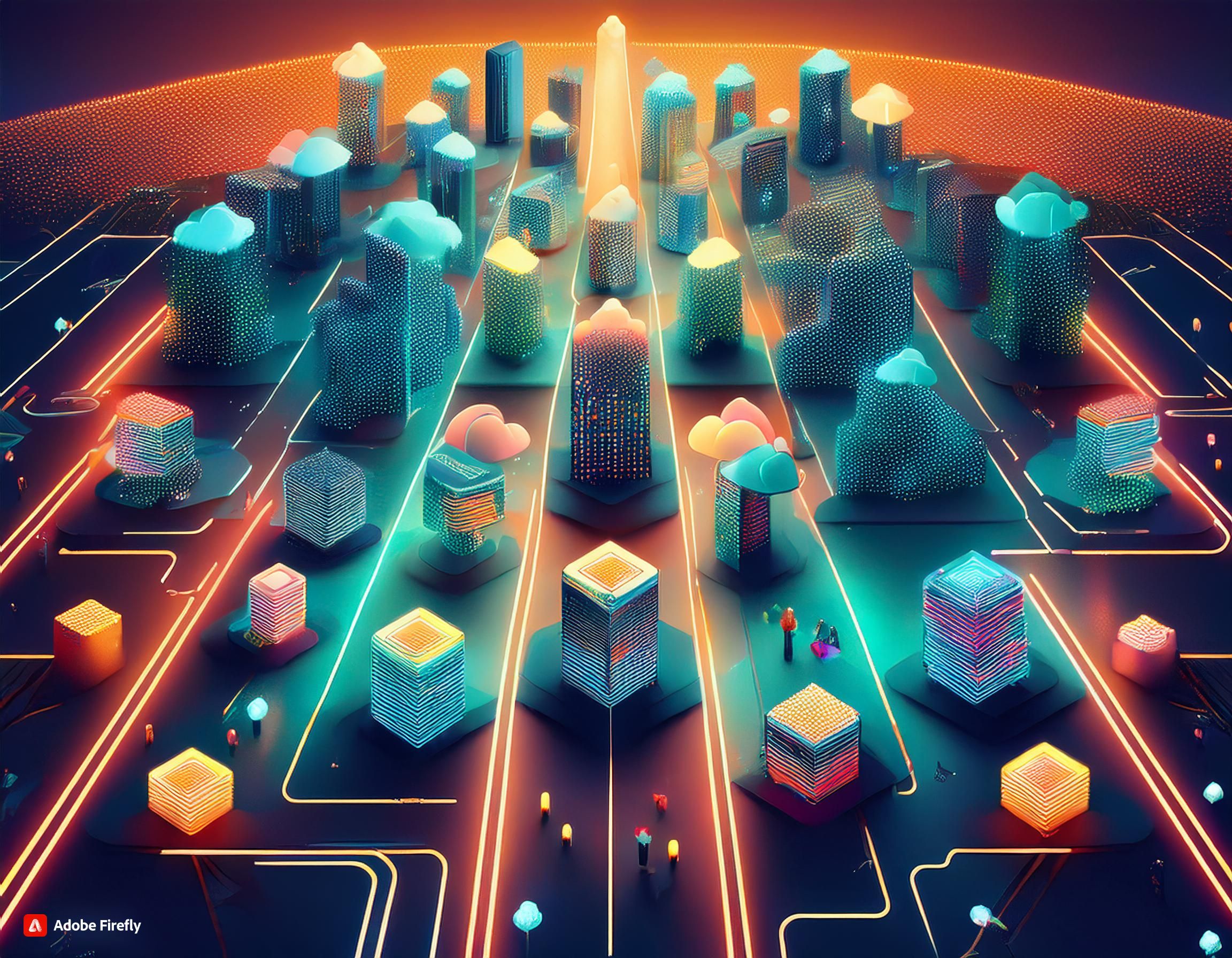
October 17, 2024
Unlock the Power of Your Data with RAG using Vertex AI and Elasticsearch
Unlock your data's potential with RAG using Vertex AI and Elasticsearch. This blog series covers data ingestion into Elasticsearch for a robust knowledge base for creating advanced RAG based search applications.
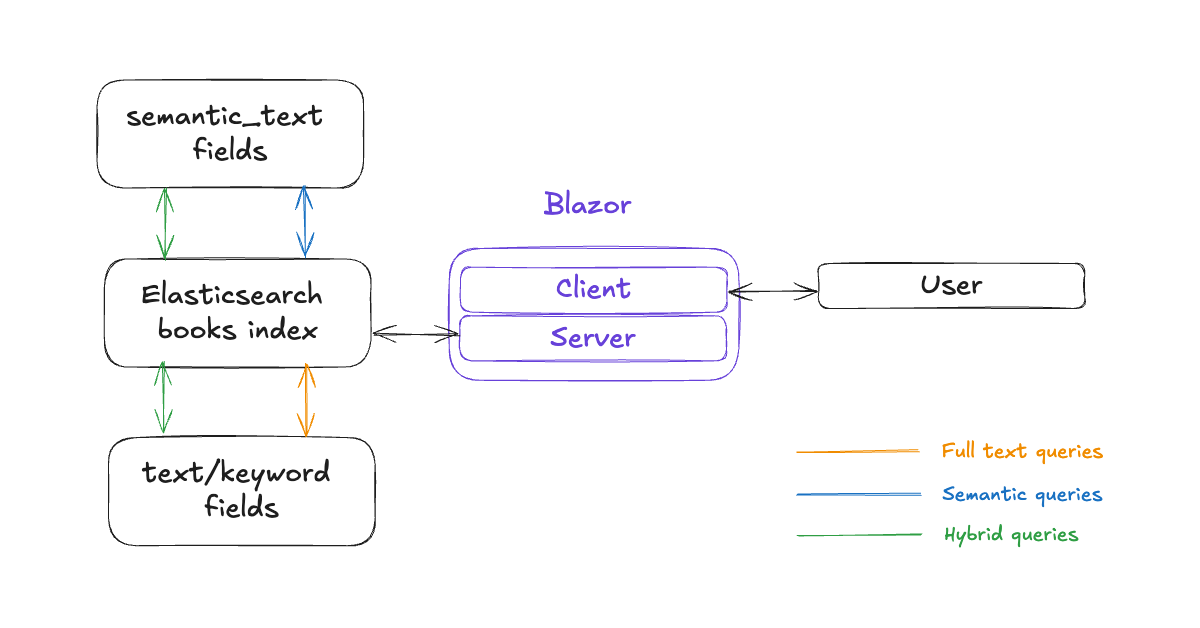
October 9, 2024
Building a search app with Blazor and Elasticsearch
Learn how to build a search application using Blazor and Elasticsearch, and how to use the Elasticsearch .NET client for hybrid search.
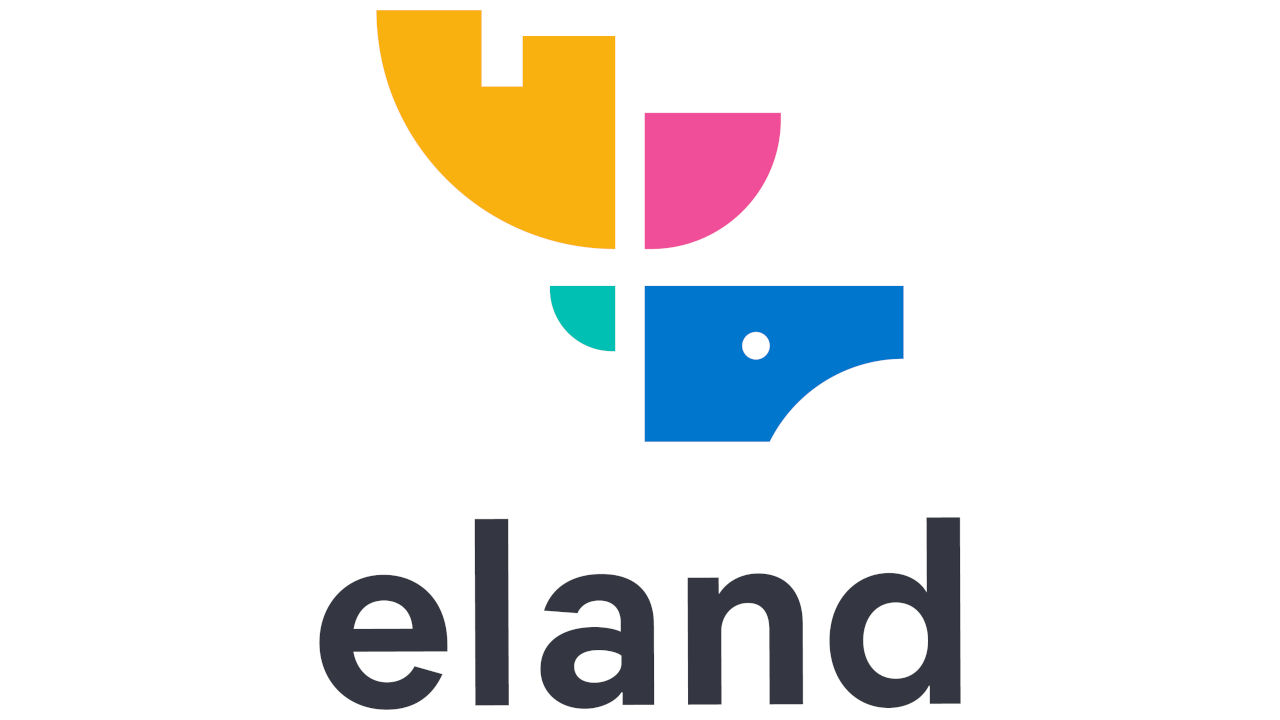
October 4, 2024
Using Eland on Elasticsearch Serverless
Learn how to use Eland on Elasticsearch Serverless
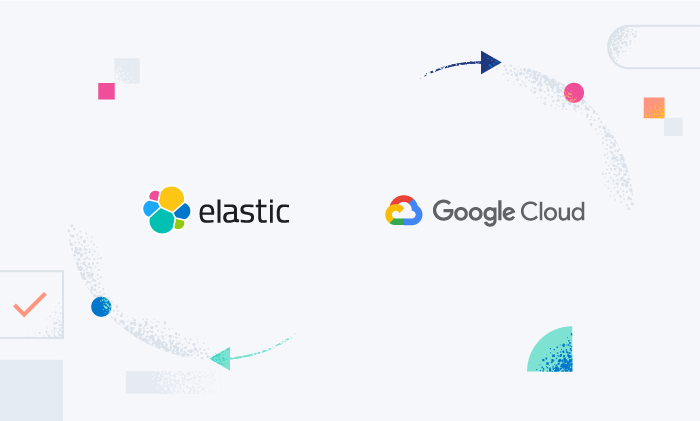
September 27, 2024
Vertex AI integration with Elasticsearch open inference API brings reranking to your RAG applications
Google Cloud customers can use Vertex AI embeddings and reranking models with Elasticsearch and take advantage of Vertex AI’s fully-managed, unified AI development platform for building generative AI apps.
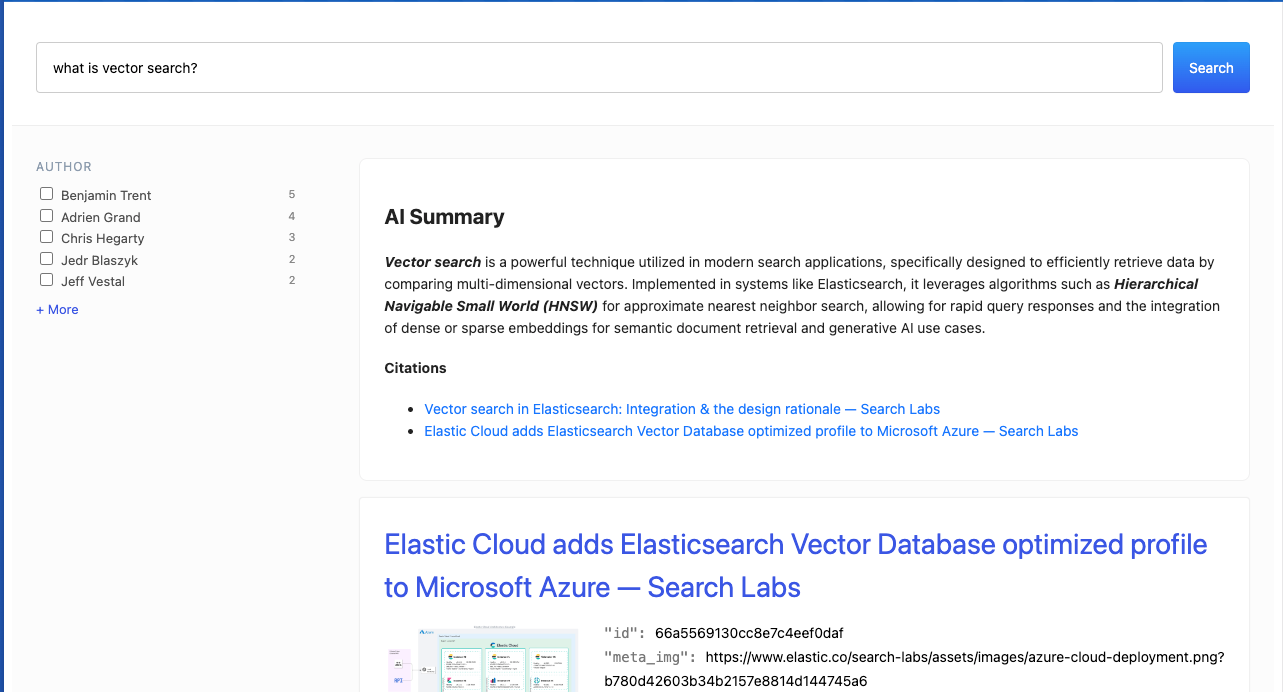
September 26, 2024
Adding AI summaries to your site with Elastic
How to add an AI summary box along with the search results to enrich your search experience.